COeXISTENCE is an ecosystem to experiment with future Urban Traffic Systems, where routing decisions are simulatenously made by humans and autonomous vehicles.
We want to understand the future of Urban Mobility and foresee what happens when our cities are shared with autonomous, intelligent robots - competing with us for limited resources.
- We demonstrated the novel phenomena on simple topologies here
- We created the dedicated MARL environment for our experiments paper and repository on GitHub
- We have some preliminary results from EWRL
- We identified issues with convergence and reported them here
For updates and new contributions you may consult our GitHub or follow us on social media.
For collaborations, please contact us or simply start contributing on our repos.
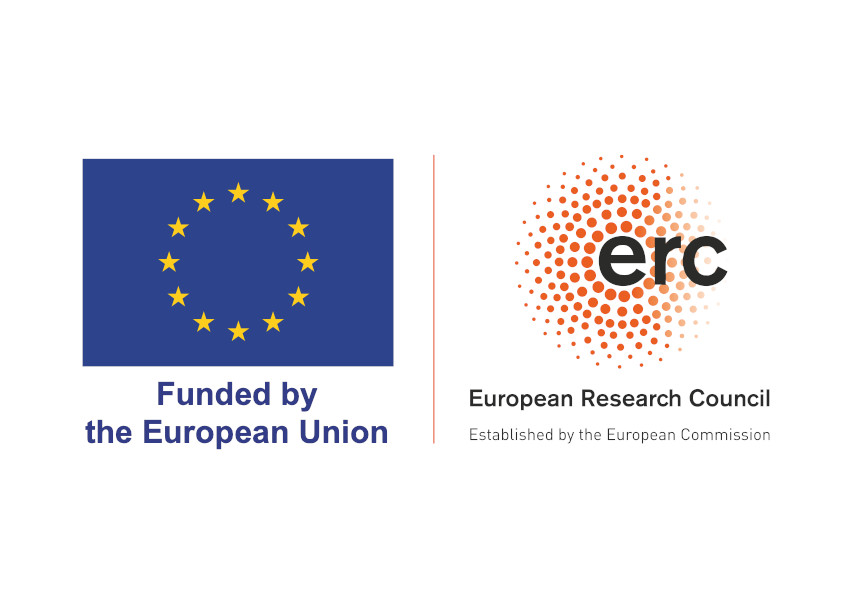
Vacancies
Our team is happily full at the moment, yet we are always happy to collaborate.
Nonetheless, we welcome Master Students, Visiting Professors (funded short term visits) or prospective PhD students in this project’s ecosystem.
Feel free to reach us out to understand more about opportunities at coexistence@uj.edu.pl
Disclaimer: Funded by the European Union. Views and opinions expressed are however those of the author(s) only and do not necessarily reflect those of the European Union or European Research Council Executive Agency (ERCEA). Neither the European Union nor the granting authority can be held responsible for them.
Funding acknowledgement: This project has received funding from the European Research Council (ERC) under the European Union’s Horizon Europe research and innovation programme (grant agreement No 101075838).
Publications linked to the project
-
RouteRL: Multi-agent reinforcement learning framework for urban route choice with autonomous vehicles
Akman, Ahmet Onur,
Psarou, Anastasia,
Gorczyca, Łukasz,
Varga, Zoltán György,
Jamróz, Grzegorz,
and Kucharski, Rafał
arXiv preprint arXiv:2502.20065
2025
RouteRL is a novel framework that integrates multi-agent reinforcement learning (MARL) with a microscopic traffic simulation, facilitating the testing and development of efficient route choice strategies for autonomous vehicles (AVs). The proposed framework simulates the daily route choices of driver agents in a city, including two types: human drivers, emulated using behavioral route choice models, and AVs, modeled as MARL agents optimizing their policies for a predefined objective. RouteRL aims to advance research in MARL, transport modeling, and human-AI interaction for transportation applications. This study presents a technical report on RouteRL, outlines its potential research contributions, and showcases its impact via illustrative examples.
-
Autonomous Vehicles Using Multi-Agent Reinforcement Learning for Routing Decisions Can Harm Urban Traffic
Psarou, Anastasia,
Akman, Ahmet Onur,
Gorczyca, Łukasz,
Hoffmann, Michał,
Varga, Zoltán György,
Jamróz, Grzegorz,
and Kucharski, Rafał
arXiv preprint arXiv:2502.13188
2025
Autonomous vehicles (AVs) using Multi-Agent Reinforcement Learning (MARL) for simultaneous route optimization may destabilize traffic environments, with human drivers possibly experiencing longer travel times. We study this interaction by simulating human drivers and AVs. Our experiments with standard MARL algorithms reveal that, even in trivial cases, policies often fail to converge to an optimal solution or require long training periods. The problem is amplified by the fact that we cannot rely entirely on simulated training, as there are no accurate models of human routing behavior. At the same time, real-world training in cities risks destabilizing urban traffic systems, increasing externalities, such as CO2 emissions, and introducing non-stationarity as human drivers adapt unpredictably to AV behaviors. Centralization can improve convergence in some cases, however, it raises privacy concerns for the travelers’ destination data. In this position paper, we argue that future research must prioritize realistic benchmarks, cautious deployment strategies, and tools for monitoring and regulating AV routing behaviors to ensure sustainable and equitable urban mobility systems.
-
Social implications of coexistence of CAVs and human drivers in the context of route choice
Jamróz, Grzegorz,
Akman, Ahmet Onur,
Psarou, Anastasia,
Varga, Zoltán György,
and Kucharski, Rafał
Scientific Reports
2025
Suppose in a stable urban traffic system populated only by human driven vehicles (HDVs), a given proportion (e.g. 10 %) is replaced by a fleet of Connected and Autonomous Vehicles (CAVs), which share information and pursue a collective goal. Suppose these vehicles are centrally coordinated and differ from HDVs only by their collective capacities allowing them to make more efficient routing decisions before the travel on a given day begins. Suppose there is a choice between two routes and every day each driver makes a decision which route to take. Human drivers maximize their utility. CAVs might optimize different goals, such as the total travel time of the fleet. We show that in this plausible futuristic setting, the strategy CAVs are allowed to adopt may result in human drivers either benefitting or being systematically disadvantaged and urban networks becoming more or less optimal. Consequently, some regulatory measures might become indispensable.